“Last time, on the Future Fellows blog…” 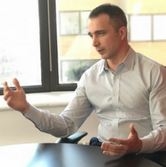
I hope we didn’t keep you in suspense for too long after our previous blog on How a Catastrophe Model Works. Today we have the second part of my interview with Beach Group’s Head of Analytics in North America and host of the Not Unreasonable podcast, David Wright, ACAS, CFA. Without further ado, this is
Part 2: Being a Catastrophe Modeler.
Future Fellows: What does your average workday look like?
David Wright: I don’t have an average day. In terms of analytics, I can tell you what an average analytically focused day for me would be. That usually winds up being trying to figure out how we answer a complicated question from a client. The basic stuff gets done pretty easily by our team, and that is where you have a basic catastrophe renewal, and you bring in the data, and you develop the loss estimates for the different layers of the catastrophe program, and we can use that to advise the client on what the pricing should be or how they should restructure the program. The problems that make it to my desk tend to be much more complicated. I tend to formulate strategies for answering questions, for example, “How do we do a takeout of policies from a state insurer?” Or I’ll have a portfolio optimization problem, or a client wants to move into a new state, or client wants to price out some other kind of change in their policy. Or there’s an error somewhere in a model, and we don’t know where it is, so we have to try to dig into the underlying information and try to figure out how to fix a problem that we can’t easily observe. It comes down to the harder problems both analytically and from a process perspective. We’re trying to be creative, on a good day, to solve a problem that isn’t something we do every day.
FF: What non-actuarial roles exist on your team? A meteorologist, for example?
DW: We have at times employed a meteorologist. We have three different groups:
- People who have a research background: That would be people who have a PhD in some kind of physical science. That would give them insight into how catastrophes happen and how catastrophe models work.
- People with programming expertise: They would be people who are building systems and tools for us, who can make the process more efficient, who can automate aspects of what we do, who have a real deep understanding for how to realize the analytical insight of an actuary or a sophisticated analyst.
- People who are really good with data. This would be kind of the pure cat modeling role, taking in dirty raw data and turning them into import files that can be input into the model and can be a generalist about execution under the direction of some other resource in the company.
FF: And so then the actuary role…?
DW: The actuary is more of an analytical quarterback. They would be somebody who understands what needs to happen to answer a question or can ask the right question and can be a leader on the team. The actuaries don’t get involved in every catastrophe account, but they’re the ones who have to answer some of the more difficult problems, where we need to understand the underlying mathematics of diversification, the underlying mathematics of the distribution that make the cat models run.
FF: What events do you typically model? What would you consider a “normal” catastrophe?
DW: Hurricanes are big. We do a lot of non-natural peril modeling in the rest of the organization, so we model portfolios, which can be impacted by casualty risks, by systemic losses, industry events. For natural peril modeling, hurricane and I would say winter storms and hail and tornado losses. Hurricane – like I was saying before – is a kind of loss that is modeled pretty well [by vendors], or at least it would be hard for us to come up with something that’s better.
Tornado, hail, and winter storm models don’t tend to be as good. There’s tons of data on winter storms, tornados, and hail—those events happen every single year. And yet still the vendor models are pretty inaccurate in their ability to calculate these losses and produce some kind of prediction or estimate that matches the historical record. I think that the reason for this is that hurricanes and earthquakes are pretty simple physical phenomena…and models are ground up estimates of loss, so they start in the physical reality, which is that the ground shakes, or the wind blows. A hurricane is a pretty contained and simple system, and so there’s some fairly simple math that underlies the difference in wind speed between the eye wall and 10 km out from the storm. You can model that! You can be pretty good at modeling that, actually.
A winter storm, or a hail storm, or a tornado is way harder to model, because they’re very local…a tornado can hit one building, everything else around it is totally fine, but that building is destroyed. So you can have a $20M loss if you wipe out a single building and it can be a $0 loss if it lands 15 feet to one side or the other. That’s an incredibly volatile physical phenomenon, so in those cases, the vendor models aren’t as strong, so you do have to come up with your own models, and we do a lot of that.
A lot of what my organization is called upon to do is to try to come up with answers to problems that aren’t as easily answered with the “standard toolbox.” So we build a lot of frequency models. The vendor models tend to be less good at modeling high frequency events. Those would be small storms, small hurricanes, winter storms, tornado, hail, and that’s said to be the domain of the actuary, and looking at the experience and thinking hard about how you might project a future claims cost based on prior experience. That’s all done outside of the model, so we do an awful lot of that.
FF: It sounds like you do a lot of the higher frequency stuff. Have you ever done anything really unusual, like super low frequency? What sort of challenges were presented by the atypical nature of that event?
DW: Yeah, we built a model years ago trying to estimate the cost of class action law suits for directors and officers insurance for a few clients. We built this model—we partnered up with Stanford University, with their law department, with a guy named Joe Grundfest. He’s a professor and a well-known individual in the securities class action world. That was modeling something where we had one data point, which was the mountain of class action lawsuits that came out of the 2001 stock market crash. A few years after that, we had the data set of securities class action lawsuits, and we [built] a model that tried to predict the class action frequency and severity for a future event.
What we didn't wind up building was a frequency model because there was only one event in the past. What it wound up actually being was just a severity model and a very simple frequency assumption, so something like once every 10 years, once every 20 years, and then a severity model which was much more sophisticated because we had a lot more data on “given that there’s a loss, what does that loss look like?” We did a good job of predicting the 2008 crisis, actually, how big that was – or wasn’t, because it didn’t turn out to be that bad in that industry – so we felt pretty good about it.
FF: When you do build your own models, what programs do you use? Do you have your own modeling software or do you just use R?
DW: R is on the list, for sure. If I’m writing code, I tend to use Python; I prefer that as a programming language, but R is on the list. VBA we’ll use for Excel. SQL if it’s simple enough that you can build it, if it’s a big data set and it’s simple enough to write SQL code, we can do that. The vast majority of the modeling that we do, though, is just straight-up Excel spreadsheets. Most actuarial data sets – I call them “medium data,” as opposed to “big data” – are small enough to fit in a spreadsheet, and if they’re small enough to fit in a spreadsheet, you’re better off putting it in a spreadsheet. The key thing that you lose when you move off of Excel is the ability to communicate it.
One of the things that we value a lot here is when we build something, we want to be able to hand it to somebody and have that person – being a counterparty, a client, a reinsurer, or somebody else – actually be able to open it and look at it and decide for themselves whether they think it’s right or not. So we try to be very transparent with the things that we build, so that’s why we prefer simple tools that are either open source or ubiquitous in the case of Excel and VBA and SQL, and that almost anybody can look at it and use it and validate it for themselves, make changes.
We’ve built models for clients where we hand it over to them and they incorporate it into their own core process, and it becomes part of their organization; we do that all the time. They might come back to us to help them tweak it later on, but we built it for a purpose and it tends to be highly customized and not really useful for anything else, and so once we build it, we might as well give it to the person we built it for if they choose to use it. So if we can at all help it, we use the most widely available tools that we can.
FF: Do you have any advice for an aspiring catastrophe modeler?
DW: A lot of times I think actuaries can get frustrated with the standard actuarial career path. I’ve come across this a few times in hiring people, where you’ll have folks who’ve kind of stopped taking exams because they’re not necessarily doing casualty actuarial work. I think that particularly the preliminary exams are an excellent training ground for the math that sits underneath the cat models, and I think at least finish those exams, because that’s going to give him or her a great advantage in delivering value to any kind of organizational process. So if you’re willing and able to go through the exam process, you can become an extremely valuable catastrophe modeler.
Learn programming for sure. I think that one of the biggest weaknesses of the CAS syllabus has been its lack of formal programming training. They’re getting there now with some of the GLM training that you take in the new exams, and there are more GLMs in the upper level exams, and I think that’s great. I think that programming training via some scripting language, or Python or R, or even VBA or SQL, I think that’s going to be a critical tool, for the actuary generally, and especially for the actuary cat modeler. Those are the folks who are interacting with data sets that are very large and need to use often some other kind of tool that’s not Excel and that you need separate training on.
Please log in to leave your comments or questions.